These days, any mention of cybersecurity solutions will inevitably lead to a conversation involving artificial intelligence (AI). That’s because the marketing of many next-generation cybersecurity tools and solutions places AI front and center as the main line of defense. This growing attention to AI is certainly affecting cybersecurity decision-makers—71% of businesses plan on investing in AI cybersecurity tools in 2019, according to a new report.
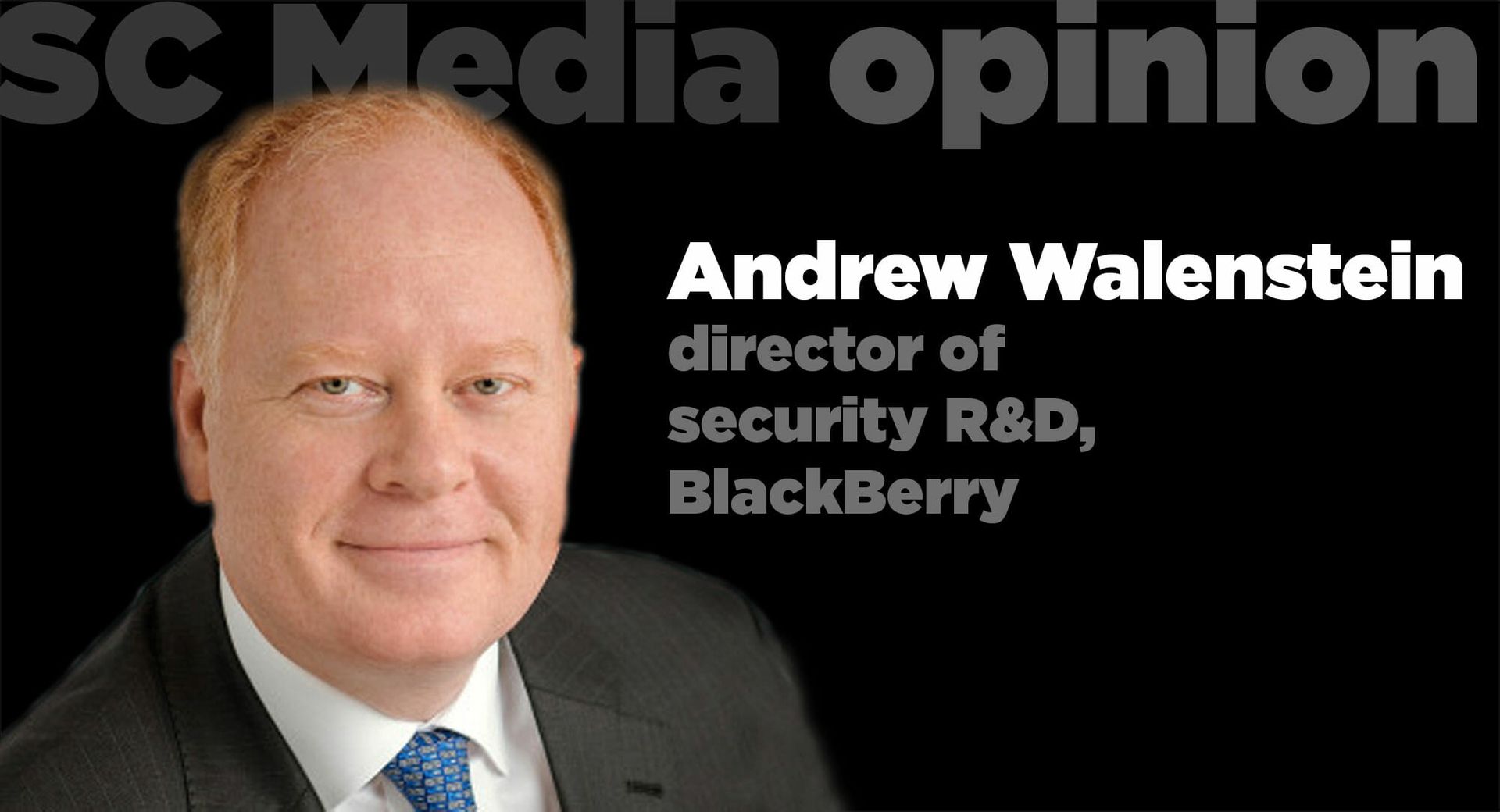
At this dizzying pace, plenty are finding themselves a little lost when it comes to understanding AI and what it is. How is AI different than machine learning (ML)? How do AI-powered security products work? How do I know which is best?
Those questions and many others like them are posed quite often. And while they’re certainly legitimate questions to ask, it’s moot to address them without first understanding what AI is and isn’t. There’s tons of misinformation out there about this very subject, so let’s start with a few facts about AI that we’re finding most people simply aren’t aware of yet.
Something that surprises many people is that AI isn’t some new kid on the block. Recently “neural network” techniques have become extremely popular, fostering the perception that they’re shiny and new. But neural networks have been around for more than half a century, and one of the first commercial neural networks for anti-malware was over 20 years ago! It protected – get this – floppy disk boot sectors in the age of Windows 98.
Another thing that seems to come as a surprise is just how many different places ML is found helping protect systems. This might be due to people reacting to the “machine” part of ML. In reality, ML is just another form of learning from examples—a concept everyone can understand. So, whether it’s a human or machine that’s learning to perform a task, all that matters is the level of sophistication and expertise that results.
A good example is the predictive keyboard on your smartphone. It has a little ML engine in it that reads what you type and learns from your typing style to predict what you might say next—or at least what you intend to say next. As you feed it more and more text, it can more confidently and accurately learn what you personally say and how you say it. The value is that you have your own non-human helper that can predict your speech. Instead of a predictive keyboard, if we feed the ML your typing, mousing and other activities, it can learn even more about your unique behavior, becoming an expert at recognizing you and your little idiosyncrasies.
Feeding these tools and algorithms the right data can help turn them into experts in their own right. For example, instead of text input, feed an ML-based solution malware and what results is a malware detector. Feed it network attacks and you have an IDS. These and many variations are found in network and endpoint protection platforms. It’s the first kind of application that many people think of for AI in cybersecurity, and it’s probably the most widespread and mature as of today.
Of course, doing all of this ML isn’t as simple as pointing a computer at a problem. Creating leading, world-class ML-based solutions takes more than simple tinkering. These algorithms are only as good as the data humans provide them, meaning we’re still very far off from a self-learning machine that doesn’t require input from a human in order to function. The AI-fueled apocalypse of sci-fi lore is just that—science fiction. But AI and ML-based cyberattacks and threat protection are our current reality. With malicious actors turning to AI/ML to conduct cyberattacks, it’s important we actually arm and align ourselves with these machines in order to stay safe.
Andrew Walenstein is Director of Security R&D at BlackBerry.