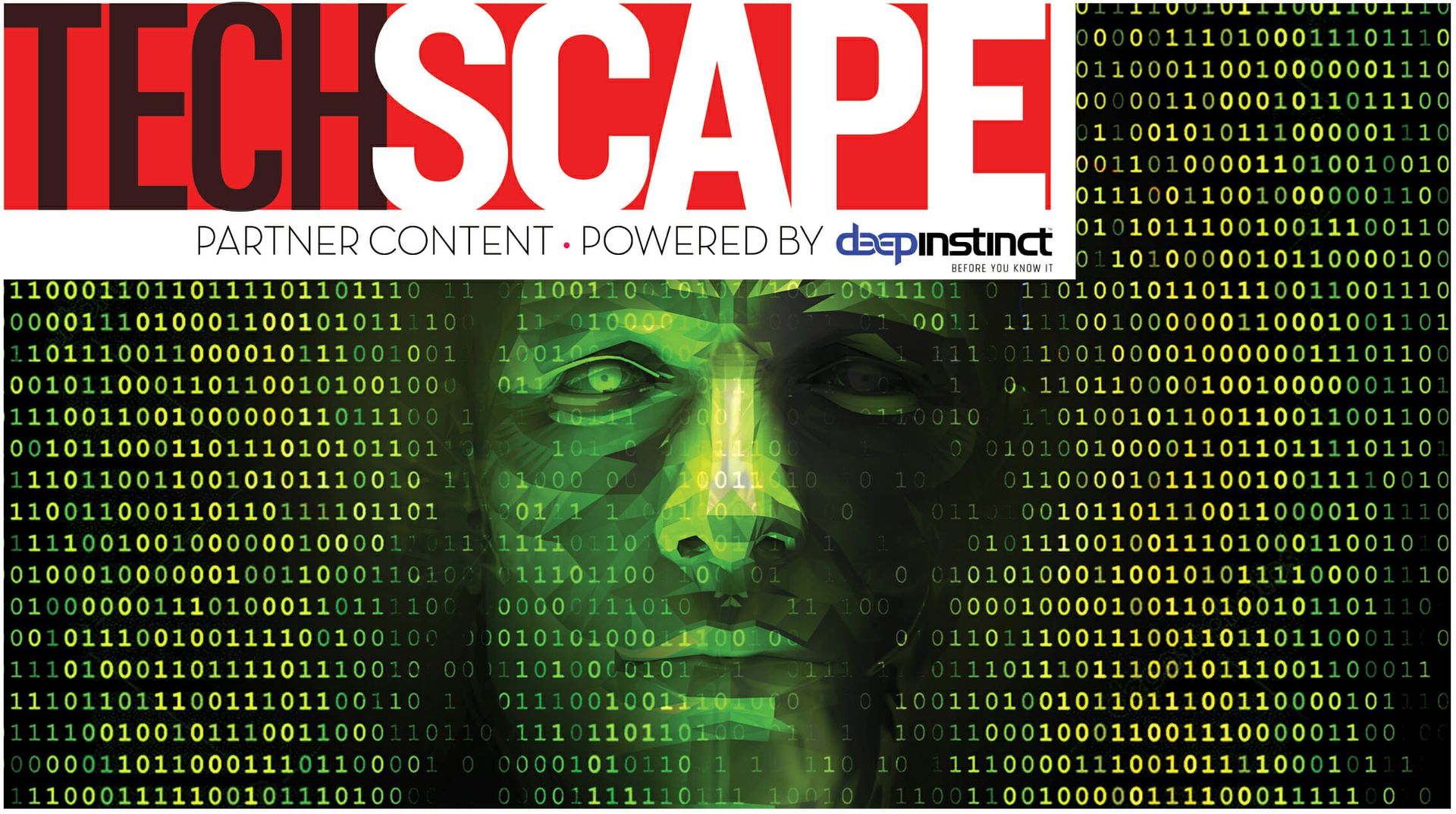
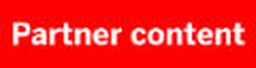
The amplified efficiency of AI means that, once a system is trained and deployed, malicious AI can attack a far greater number of devices and networks more quickly and cheaply than a malevolent human actor. Given sufficient computing power, an AI system can launch many attacks, be more selective in its targets and more devastating in its impact.
Currently, the use of AI for attackers is mainly pursued at an academic level and we have yet to see AI attacks in the wild. However, there is much talk in the industry about attackers using AI in their malicious efforts, and defenders using machine learning as a defense technology.
There are three types of attacks in which an attacker can use AI:
AI-based Cyberattacks: The malware operates AI algorithms as an integral part of its business logic. AI algorithms can be used to identify irregular user and system activity patterns, and it can be repurposed to conduct different types of attacks which are determined by the AI prediction model. For example, it can be used to increase or decrease evasion and stealth configurations, and undermine data security and integrity. An example of this is DeepLocker, demonstrated by IBM security, which encrypted ransomware to conceal the attack until the right attributes, based on a face recognition algorithm, were identified to autonomously decrypt and release the ransomware.
AI-facilitated Cyberattacks: The malicious code and malware running on the victim’s machine does not include AI algorithms, but the AI is used elsewhere in the attacker’s environment. An example of this is Info-stealer malware which uploads a lot of personal information to the C&C server, which then runs an NLP algorithm to cluster and classify sensitive information as interesting (e.g. credit card numbers). Another example of this is Spear fishing where an email is sent to the target with a facade that looks legitimate, collecting and using information specifically relevant to the attacker.
Adversarial attacks: The use of malicious AI algorithms to subvert the functionality of benign AI algorithms. This is done by using the algorithms and techniques that are built into a traditional machine learning algorithm and “breaking” it by reverse engineering the algorithm. Skylight Cyber recently demonstrated an example of this when they were able to trick Cylance’s AI based antivirus product into detecting a malicious file as benign.
Constructive AI versus malicious AI trends will continue to increase and spread across the opaque border that separates academic proof of concepts from actual full-scale attacks in the wild. This will happen incrementally as computing power and deep learning algorithms become more and more available to the wider public.
To best defend against an AI attack, you need to adopt the mindset of a malicious actor. Machine learning and deep learning experts need to be familiar with these techniques in order to build robust systems that will defend against them.
For more examples of how each of the AI types of attack have been discovered, read the full article.
Nadav Maman brings 15 years of experience in customer-driven business and technical leadership. He has a proven track record in managing technical complex cyber projects, including design, execution and sales. He has vast hands-on experience with data security, network design, and implementation of complex heterogeneous environments .
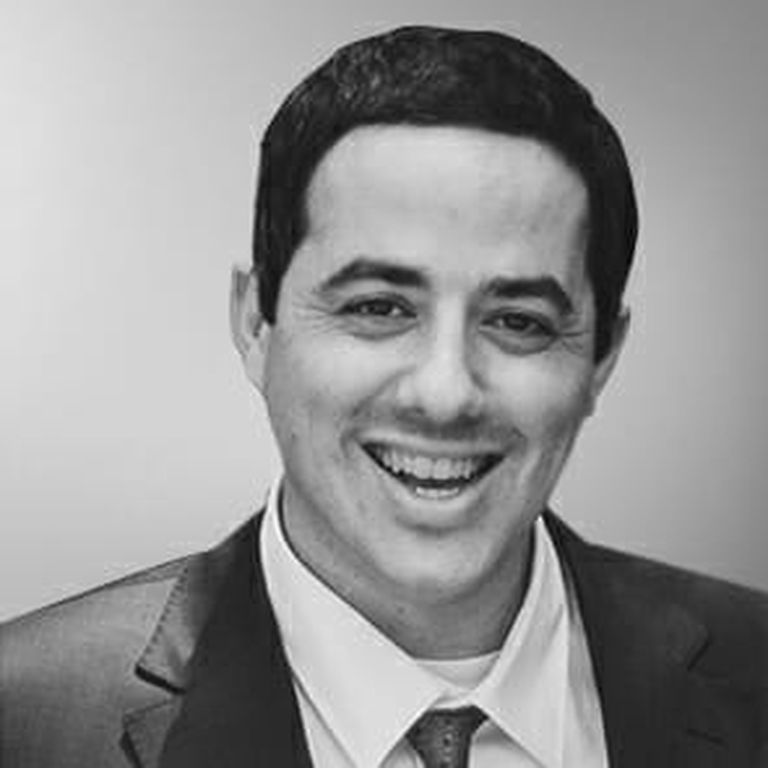